Membuat Penting Hal yang Tidak Penting dan Membuat Tidak Penting Hal yang Dianggap Penting.
Senin, 26 Oktober 2009
Custom Exponential Smoothing Models
Custom Exponential Smoothing Models
Model Type. Exponential smoothing models (Gardner, 1985) are classified as either seasonal or nonseasonal. Seasonal models are only available if a periodicity has been defined for the active dataset (see "Current Periodicity" below).
• Simple. This model is appropriate for series in which there is no trend or seasonality. Its only smoothing parameter is level. Simple exponential smoothing is most similar to an ARIMA model with zero orders of autoregression, one order of differencing, one order of moving average, and no constant.
• Holt's linear trend. This model is appropriate for series in which there is a linear trend and no seasonality. Its smoothing parameters are level and trend, which are not constrained by each other's values. Holt's model is more general than Brown's model but may take longer to compute for large series. Holt's exponential smoothing is most similar to an ARIMA model with zero orders of autoregression, two orders of differencing, and two orders of moving average.
• Brown's linear trend. This model is appropriate for series in which there is a linear trend and no seasonality. Its smoothing parameters are level and trend, which are assumed to be equal. Brown's model is therefore a special case of Holt's model. Brown's exponential smoothing is most similar to an ARIMA model with zero orders of autoregression, two orders of differencing, and two orders of moving average, with the coefficient for the second order of moving average equal to the square of one-half of the coefficient for the first order.
• Damped trend. This model is appropriate for series with a linear trend that is dying out and with no seasonality. Its smoothing parameters are level, trend, and damping trend. Damped exponential smoothing is most similar to an ARIMA model with 1 order of autoregression, 1 order of differencing, and 2 orders of moving average.
• Simple seasonal. This model is appropriate for series with no trend and a seasonal effect that is constant over time. Its smoothing parameters are level and season. Simple seasonal exponential smoothing is most similar to an ARIMA model with zero orders of autoregression, one order of differencing, one order of seasonal differencing, and orders 1, p, and p + 1 of moving average, where p is the number of periods in a seasonal interval (for monthly data, p = 12).
• Winters' additive. This model is appropriate for series with a linear trend and a seasonal effect that does not depend on the level of the series. Its smoothing parameters are level, trend, and season. Winters' additive exponential smoothing is most similar to an ARIMA model with zero orders of autoregression, one order of differencing, one order of seasonal differencing, and p + 1 orders of moving average, where p is the number of periods in a seasonal interval (for monthly data, p = 12).
• Winters' multiplicative. This model is appropriate for series with a linear trend and a seasonal effect that depends on the level of the series. Its smoothing parameters are level, trend, and season. Winters' multiplicative exponential smoothing is not similar to any ARIMA model.
Current Periodicity. Indicates the periodicity (if any) currently defined for the active dataset. The current periodicity is given as an integer--for example, 12 for annual periodicity, with each case representing a month. The value None is displayed if no periodicity has been set. Seasonal models require a periodicity. You can set the periodicity from the Define Dates dialog box.
Dependent Variable Transformation. You can specify a transformation performed on each dependent variable before it is modeled.
• None. No transformation is performed.
• Square root. Square root transformation.
• Natural log. Natural log transformation.
Langganan:
Posting Komentar (Atom)
Makan Bersama di Lombok Namanya Begibung
Halo, teman-teman! Kali ini saya mau berbagi pengalaman saya yang pernah mendapat undangan makan dari teman dalam rangka maulid nabi. A...
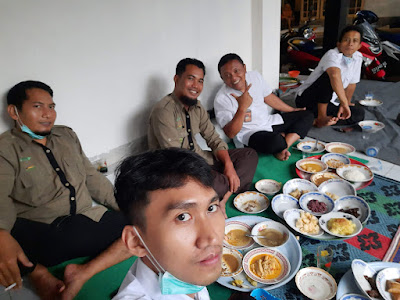
Populer, Sist/Broo
-
Sebentar lagi akan memasuki Hari Raya Idul Fitri. Tidak ada salahnya untuk mengucapkan maaf kepada semua sanak saudara, dan handai taulan. ...
-
Tugu Lawet Landmark Kebumen Ketika Saya masih di Kebumen mungkin tidak akan berpikir untuk membuat tulisan ini, tetapi ketika Saya be...
-
1. Video Optimization (SEO Optimization) Sebagai penerbit video online, berikut adalah beberapa trik tentang cara untuk mendapatka...
-
Uang merupakan alat tukar yang bisa dibawa kemana-mana. Tapi, kita kadang kesal karena uang yang kita bawa tidak rapi. Hal ini mengakibatkan...
-
Akhir-akhir ini Saya sering mendengar lagunya Barry Manilaw yang berjudul I can't smile without you , eh ternyata lagu tersebut dipak...
-
Pontianak adalah Ibu Kota Provinsi Kalimantan Barat. Letaknya berada dibagian barat Pulau Kalimantan. Kota Pontianak dibelah oleh Sungai K...
-
Kabupaten Kebumen berada di Provinsi Jawa Tengah bagian selatan. Letaknya sangat strategis karena berada pada jalur lalulintas Pantai Sela...
-
translate yang tidak mengerti silakan gunakan google translate . pilih bahasa ngapak kebumen ke bahasa Indonesia ------------------------...
-
1. Hamvee MMWV Humvee sekilas, The HMMWV (High-Mobility Multipurpose Wheeled Vehicle - atau juga disebut Humvee) adalah kendaraan multi...
-
Touring ke Waduk Wadaslintang Via Alian, (salah satu objek wisata di Kabupaten Kebumen, Jawa Tengah)Perjalanan kali ini penuh dengan petualangan, bukan hanya melelahkan secara fisik tetapi mental juga ikut ngosngosan . Tetapi kesimp...
Tidak ada komentar:
Posting Komentar
No Porn, Racism, Sadism